Rapid Evaluation, Assessment, and Learning Methodology (REALM)
Rapid Evaluation, Assessment, and Learning Methodology (REALM) are systematic monitoring and evaluation strategies that employ an expeditious approach to program improvement and design by using timely, yet data-driven, actionable evidence that supports well-informed decision-making (USAID, 2015). Utilized and developed from a number of contexts and backgrounds, REALM strategies share a set of core characteristics but differ with respect to their context, and purpose. With origins in the humanitarian global health sector, REALM was originally intended for time- and resource-sensitive contexts that demand evidence of multi-sectoral impact in a short period of time. Unlike summative evaluations which assess the impact of a program overall, REALM assesses the impact of individual program components, changes, or alternatives by gathering data, analyzing findings, and taking action over a cycle of anywhere between a few weeks to a few months. Read the GC-DWC's brief on REALM.
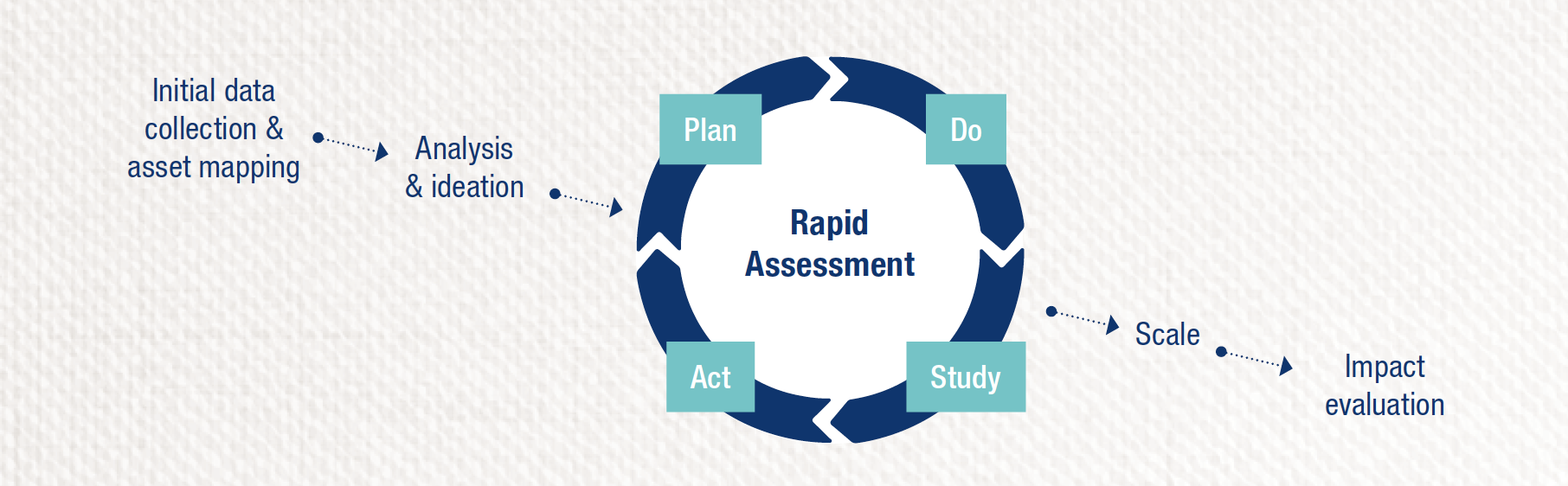
Real-time evaluations--feedback loops whereby preliminary evaluation results are shared during the fieldwork rather than several months after data collection--were developed in the 1990s by UNHCR in response to an increase in humanitarian crises and the need for quick, evidence-based evaluations (McNall & Foster-Fishman, 2007). Since then, similar methods have developed across a variety of sectors. While these methodologies differ in their approach, context, and practice they share common techniques and principles for obtaining quick yet reliable information to foster improvement:
Originating in ethnographic practices in the global health sector Rapid Assessment and Rapid Appraisal prioritize a quick understanding of the conditions preceding an intervention rather than gathering data about an ongoing activity. The goal of these REALM varieties is to generate data that helps with program design and contextualization.
A central aim of these methods is to provide immediate (real-time) feedback to help with the planning or implementing of a project or program. These variations of REALM tend to use existing implementation and performance data to make programming decisions and assess performance.
These methods have developed primarily from the technology and software development sector where the availability of user and log data allows for quickly assessing the trends in outcomes; additionally, the availability of big data in these sectors has also made Predictive Analytics possible through machine learning and looped algorithms. Typically these methods are focused on a specific activity or intervention and used to clarify uncertainty about whether or not it will have the intended impact as the project is scaled.
Used primarily in education settings in high-resource contexts, these methods emphasize using frequent and mixed methods data collection to make evidence-based changes that improve the student experience. However, improvement cycles are led by embedded school personnel and administrators rather than field researchers.
Our work at the center is focused on translating these methods into an adapted REALM strategy that can be used in the low-resource and fragile contexts where we work. Our use of REALM has highlighted key benefits to this strategy:
-
Keeps the program users/recipients at the center of the design: REALM help not only amplify the voices of those involved in or being served by the program or project but also provide community/school stakeholders with a significant degree of ownership in not only the research process but also the implementation of new and amended intervention approaches.
-
Ensures that regular feedback loops are built into data collection cycles: Because of the rapid, iterative nature of these methodologies, REALM are particularly beneficial in time sensitive and resource scarce contexts where efficiency is key. However, the rapid action that is taken based on REALM-generated data requires that feedback loops with partners and implementation staff are set up prior to data collection so that there are established ways-of-working and methods of addressing changes to the program.
-
Focused on specific program components and mechanisms: REALM allows implementers and researchers to evaluate the performance of specific program components. By obtaining frequent information and quickly producing findings, decisions can be made more rapidly than in a summative evaluation, therefore saving both time and resources by identifying effective approaches and avoiding ineffective investments
-
Appropriate for use when scaling projects: The use of REALM during a pilot phase gives a program or intervention a chance to become well developed before it is scaled. When it is scaled, therefore, the program will be more likely to succeed and require minimal alteration.
-
Appropriate for testing alternative strategies: An innovative aspect of REALM is the ability to study two or more alternative interventions approaches in a short amount of time by either trying one and then the other, or testing both simultaneously in separate groups.
-
Useful when tailoring programs to a new setting: By testing and iterating from the start, REALM helps calibrate an intervention, program, or activity to a new setting.